Lawyers are rightly proceeding with caution when integrating AI tools into their practice. The chance of factually inaccurate output is the reason for this caution. Understanding the limitations and risks of using AI models will be essential for lawyers attempting to leverage these tools. This post is a very brief summary of why factually inaccurate output occurs (i.e., hallucinations). More research should be done based on your specific use case.
It starts with the data an AI model uses during training. These data are not just random bits and pieces. They contain underlying patterns. During the training process, our AI model starts recognizing these patterns, forming a clearer picture of the underlying patterns and relationships.
Data are often represented with mathematical vectors. This might sound complex, but these are simply a format that computers can easily read and process. These vectors are pretty versatile, allowing us to input diverse types of real-world data, be it numerical, text, images, or audio, into our AI systems. So, in a sense, they act as translators, helping the model learn not only explicit facts about the world but how each fact is related.
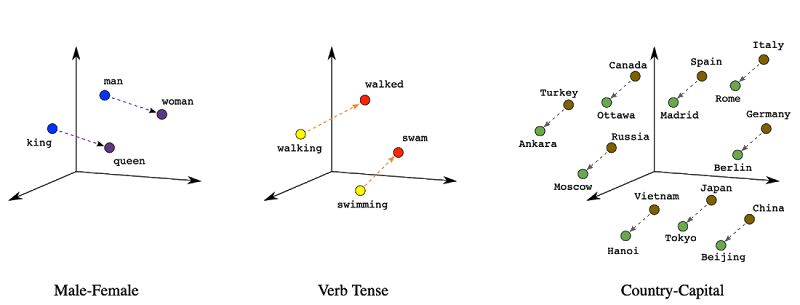
Image source: Dale Markowitz, “Meet AI’s multitool: Vector embeddings,” Google Cloud Blog, https://cloud.google.com/blog/topics/developers-practitioners/meet-ais-multitool-vector-embeddings
Hallucinations are caused by AI models working with imperfect data. One of the significant contributors to hallucinations comes from the very nature of how input data are stored in digital memory. Consider the figure (above) on the far left; how would you represent the word "princess" in this space? Well, perhaps, based on the minimal data on that chart, the word princess would be represented somewhere between woman and queen--closer to female than male, but more associated with royalty than the word "woman." The data is imperfect and the model will do its best to figure out what the word princess means. In this way, a lack of input data may create the need for a model to provide more inferences or pattern predictions without concrete input data to reference. Likewise, inaccurate input data could similarly create issues for other words in the vector space as the model tries to understand how each word is related.
Input data is the lifeblood of Artificial Intelligence. It encapsulates the extent of everything an AI model understands. Lawyers using AI tools may remedy the hallucination issue by providing accurate data relevant to their use of the tool. Without expressly telling the AI tool important facts, it may try to fill in the gaps with its best guess, creating factual inaccuracies. Courts and regulatory bodies rightly are warning about these potential risks. Indeed, as the linked article shows, even the Canadian government is providing guidance to its institutions about these risks.